标签:can multitask change code chm nts -o init sdn
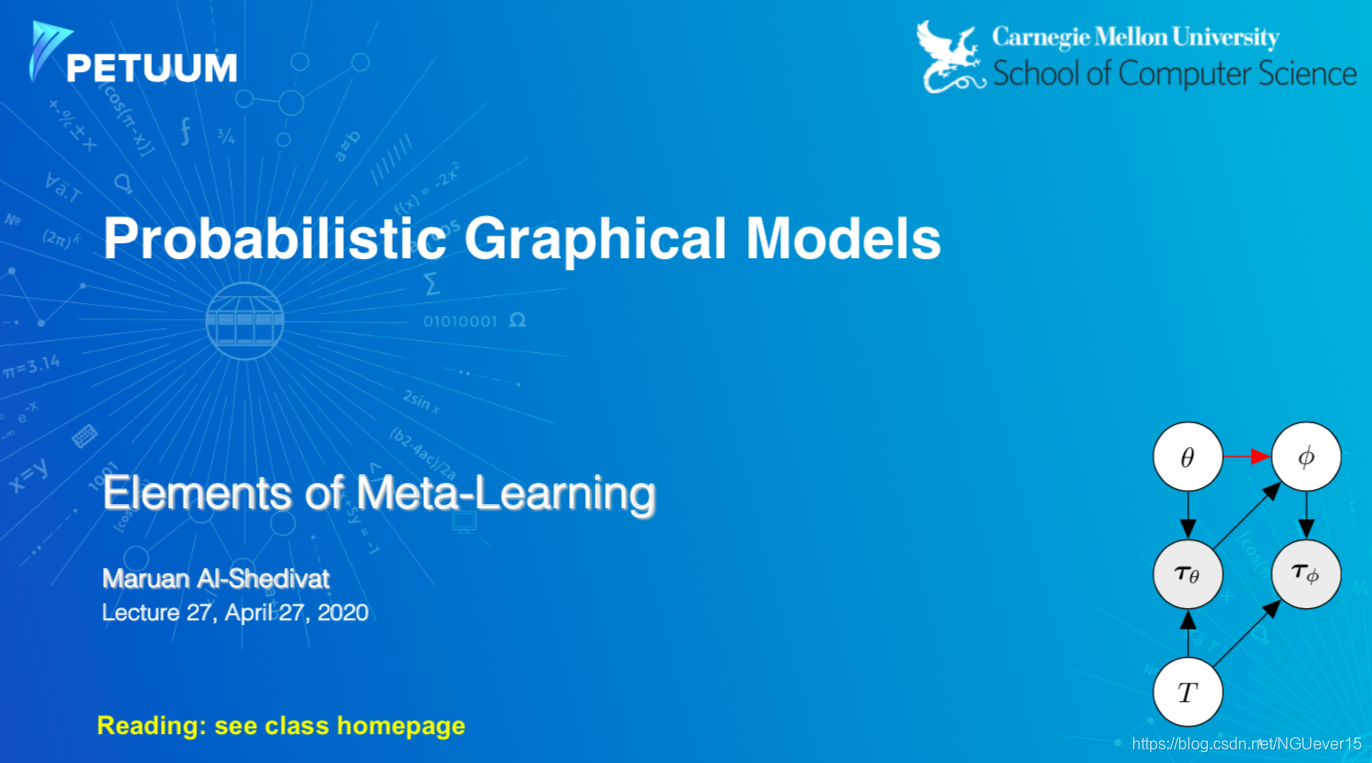
Goals for the lecture:
Introduction & overview of the key methods and developments.
[Good starting point for you to start reading and understanding papers!]
原文链接:
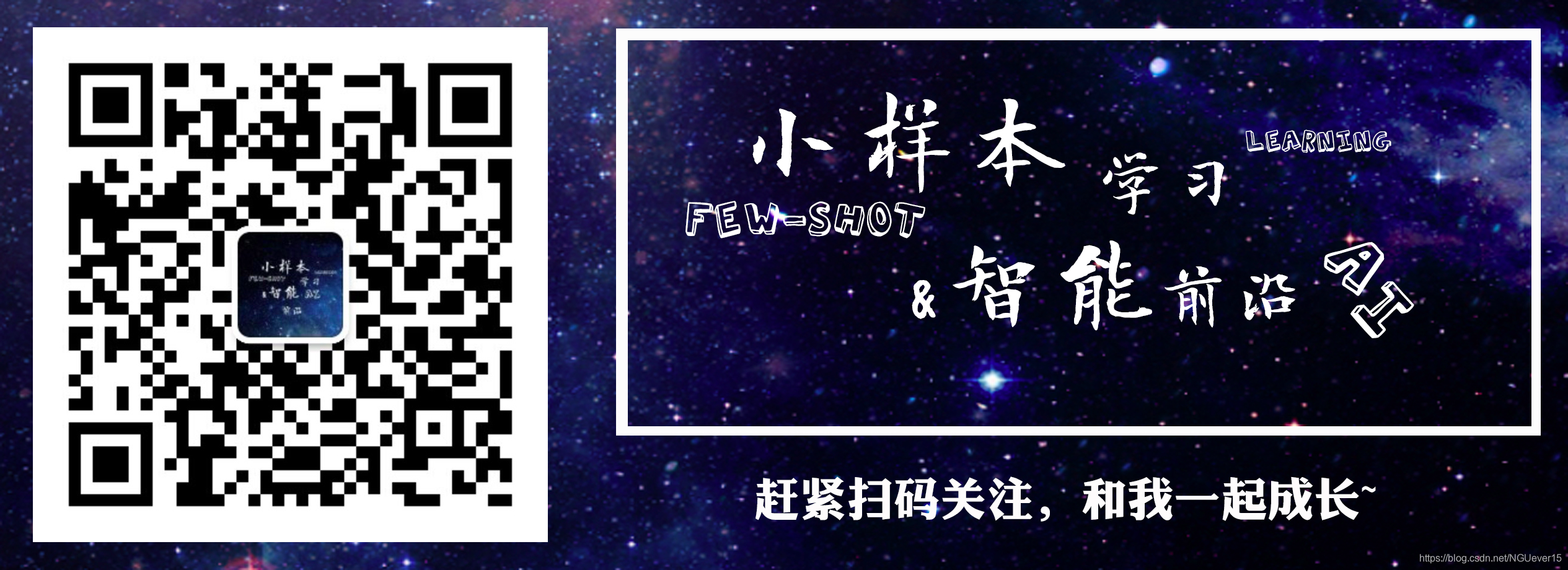
@
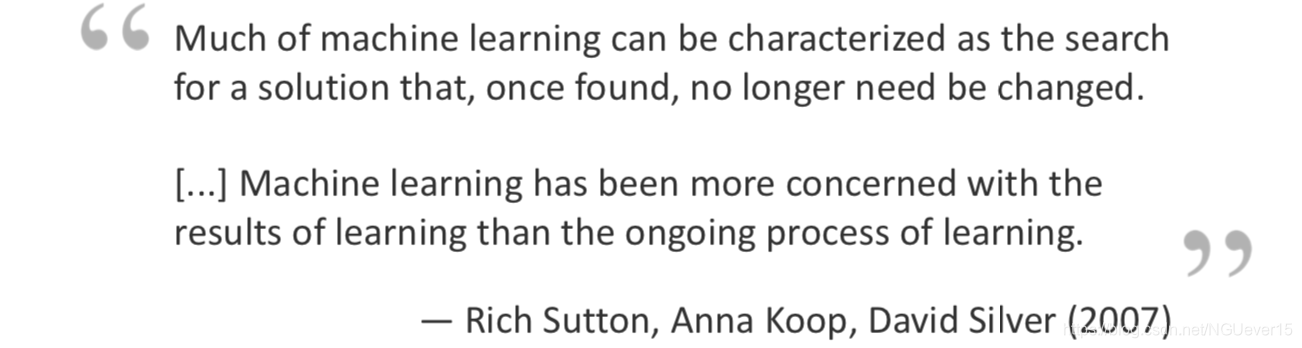
Motivation and some examples
When is standard machine learning not enough?
Standard ML finally works for well-defined, stationary tasks.
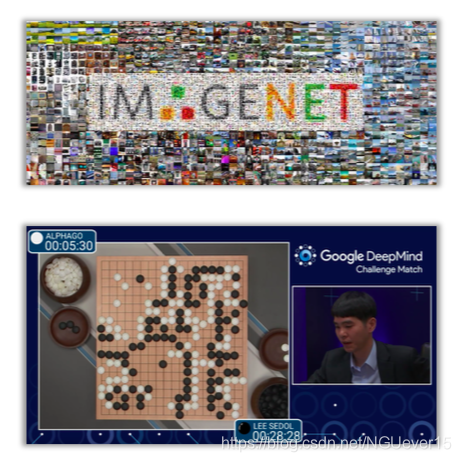
But how about the complex dynamic world, heterogeneous data from people and the interactive robotic systems?
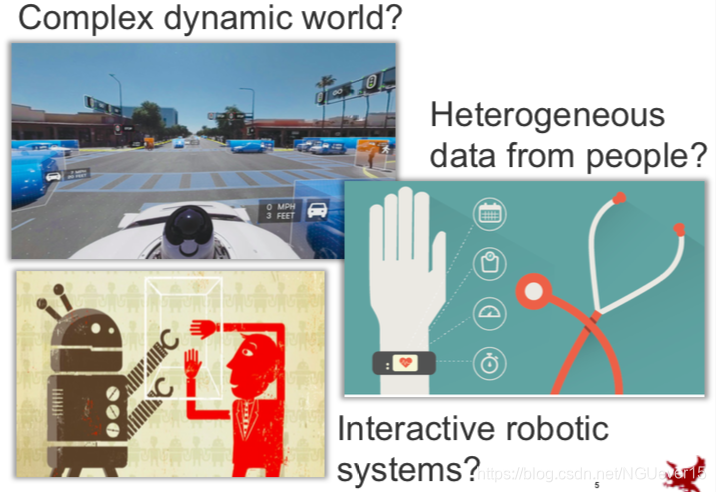
What is meta-learning?
Standard learning: Given a distribution over examples (single task), learn a function that minimizes the loss:

Learning-to-learn: Given a distribution over tasks, output an adaptation rule that can be used at test time to generalize from a task description
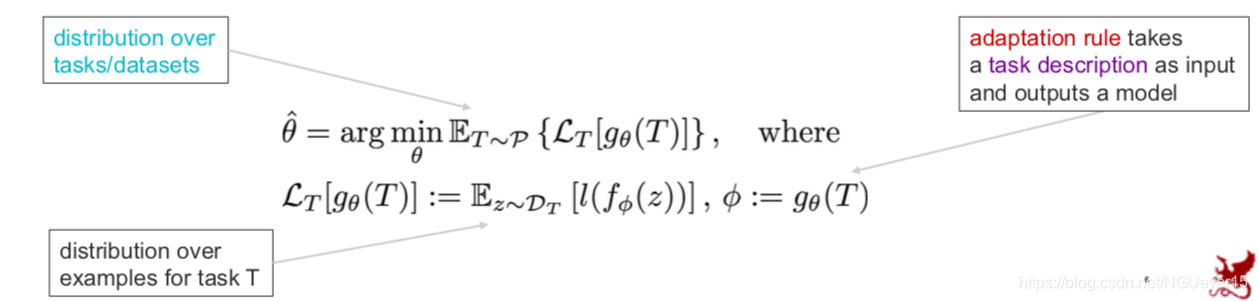
A Toy Example: Few-shot Image Classification
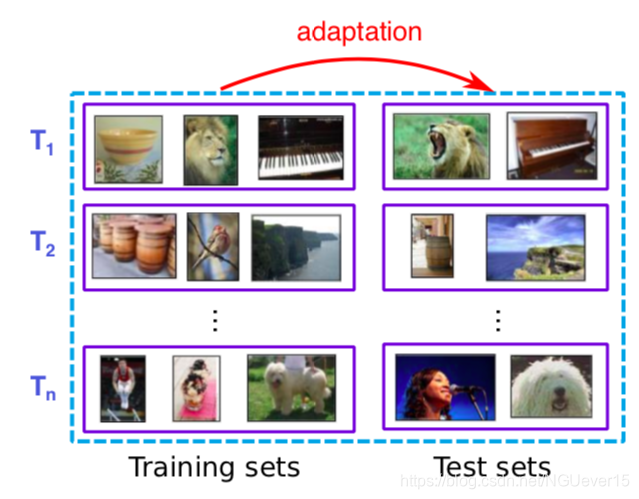
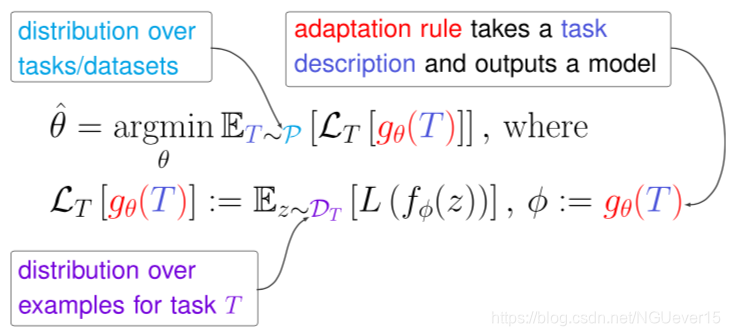
Other (practical) Examples of Few-shot Learning
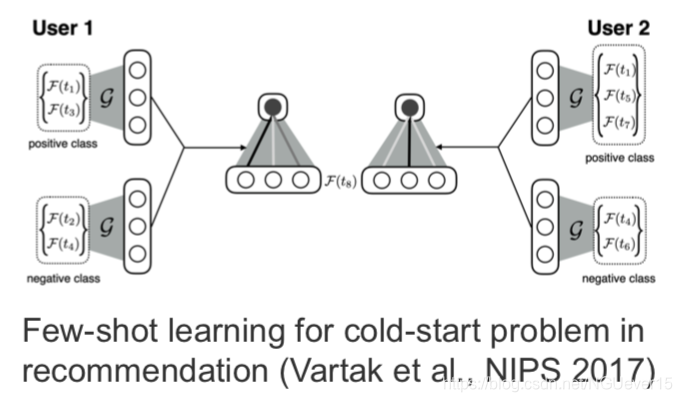
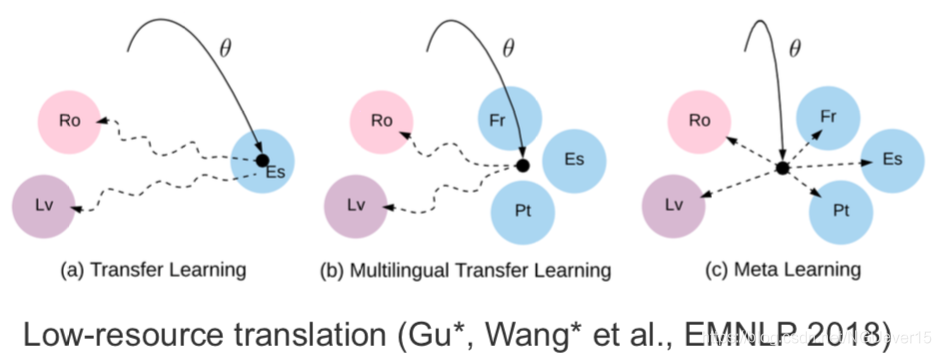
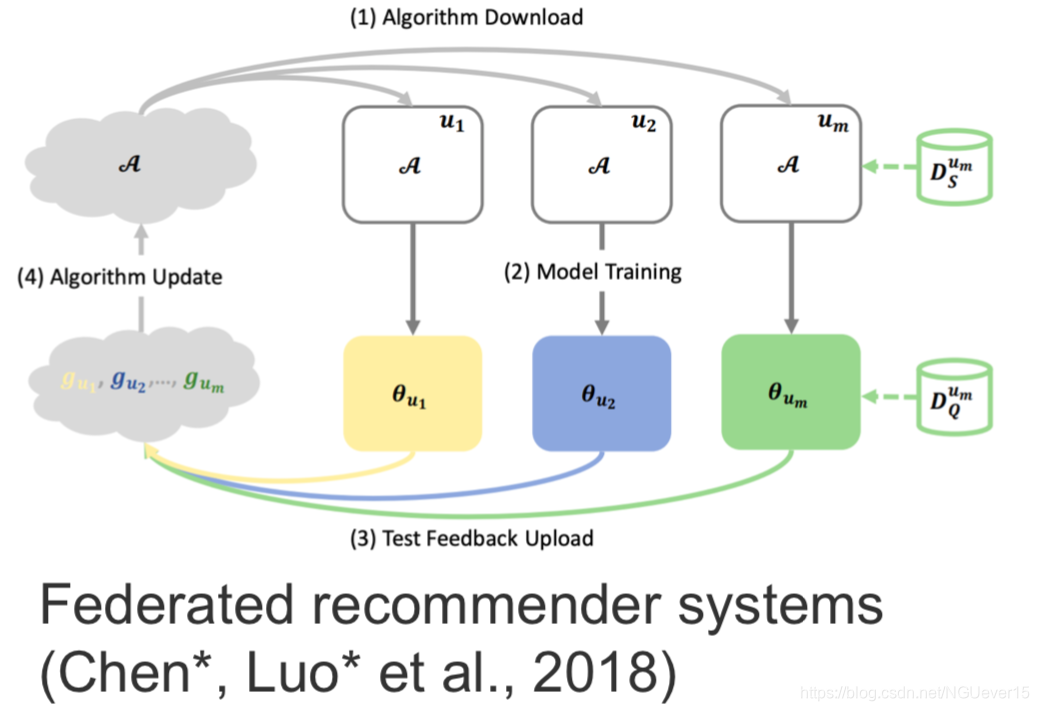
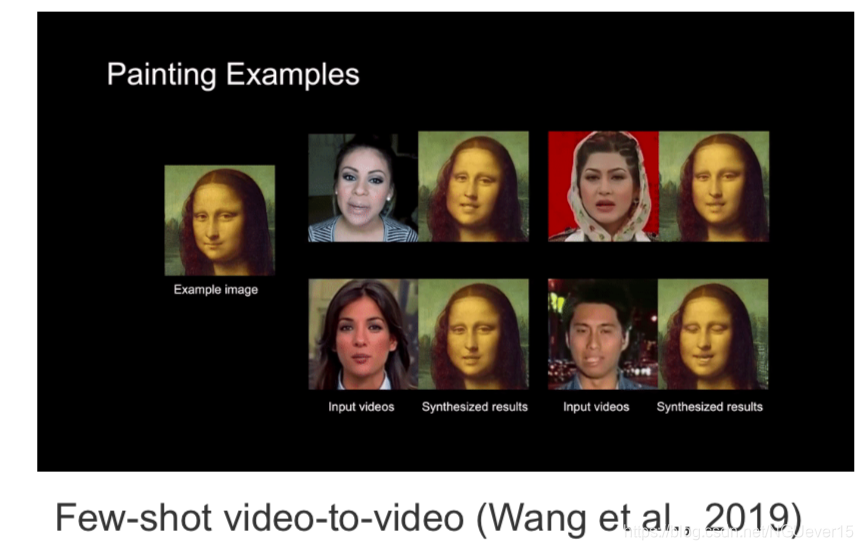
Model-agnostic Meta-learning (MAML) 与模型无关的元学习
- Start with a common model initialization \(\theta\)
- Given a new task \(T_i\) , adapt the model using a gradient step:

- Meta-training is learning a shared initialization for all tasks:

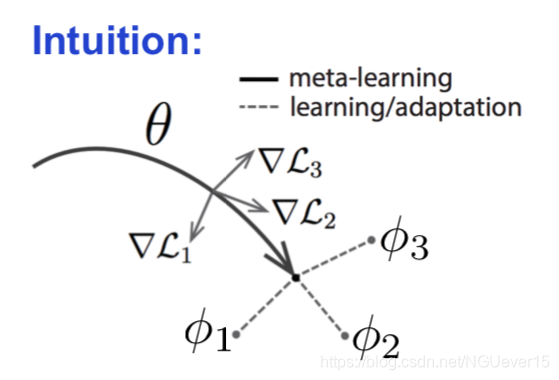
Does MAML Work?
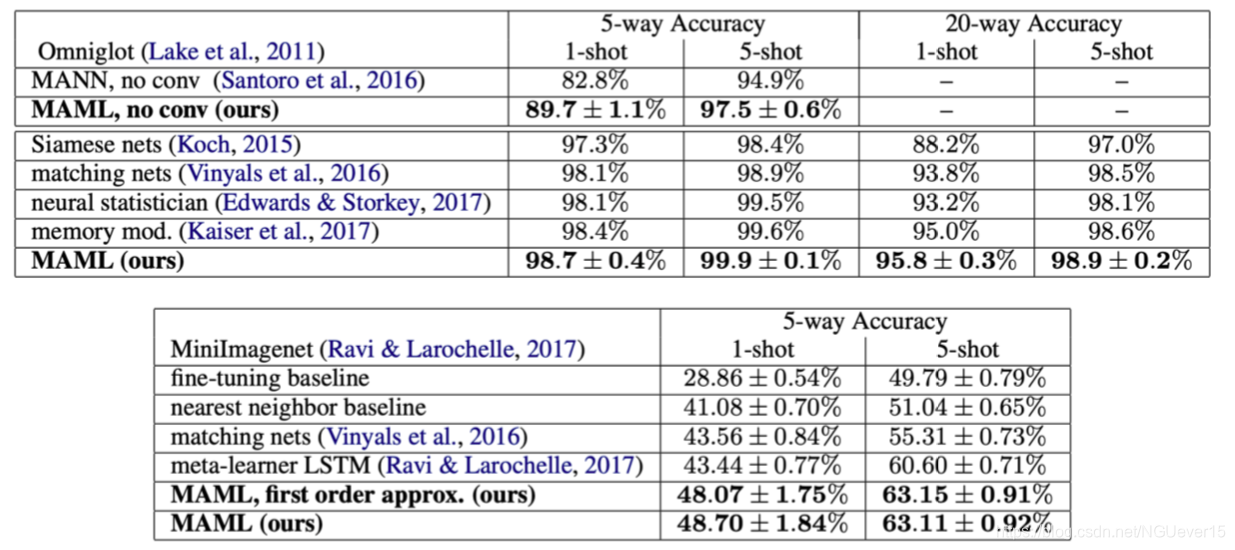
MAML from a Probabilistic Standpoint
Training points: 
testing points:
MAML with log-likelihood loss对数似然损失:

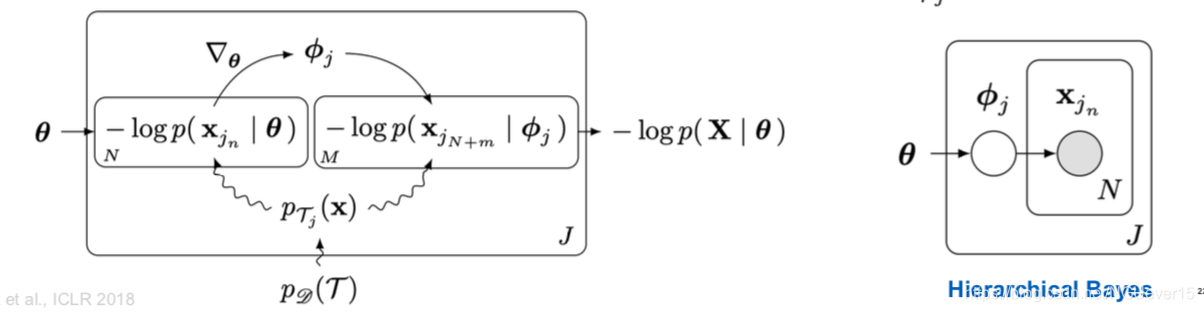
One More Example: One-shot Imitation Learning 模仿学习
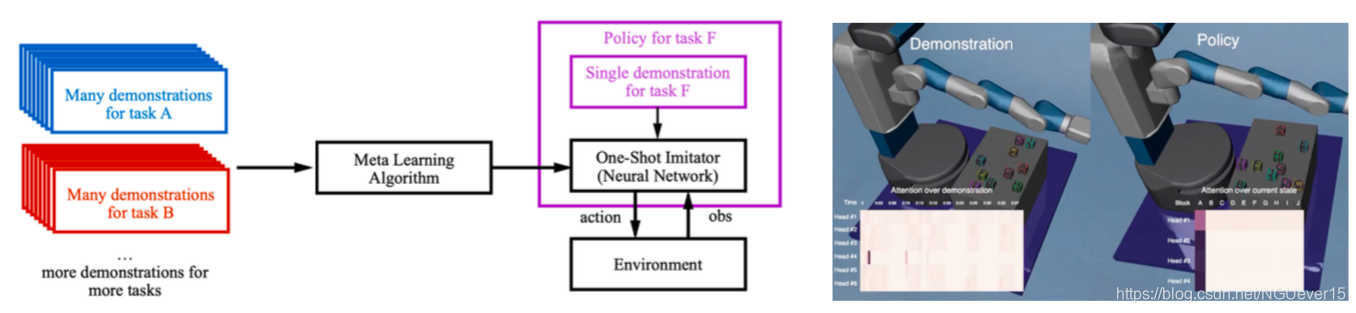
Prototype-based Meta-learning
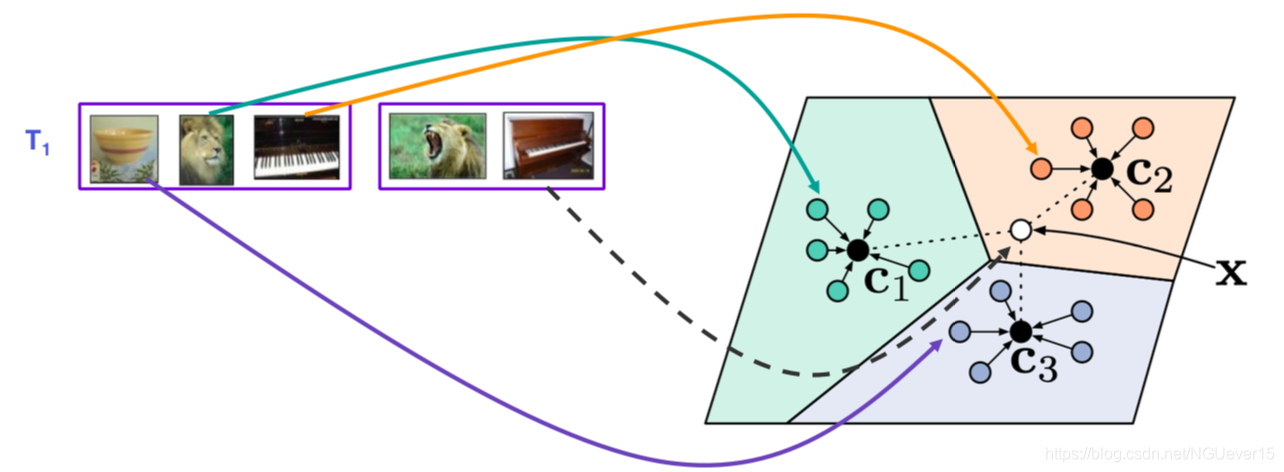
Prototypes:
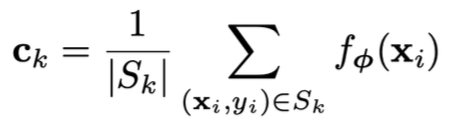
Predictive distribution:

Does Prototype-based Meta-learning Work?
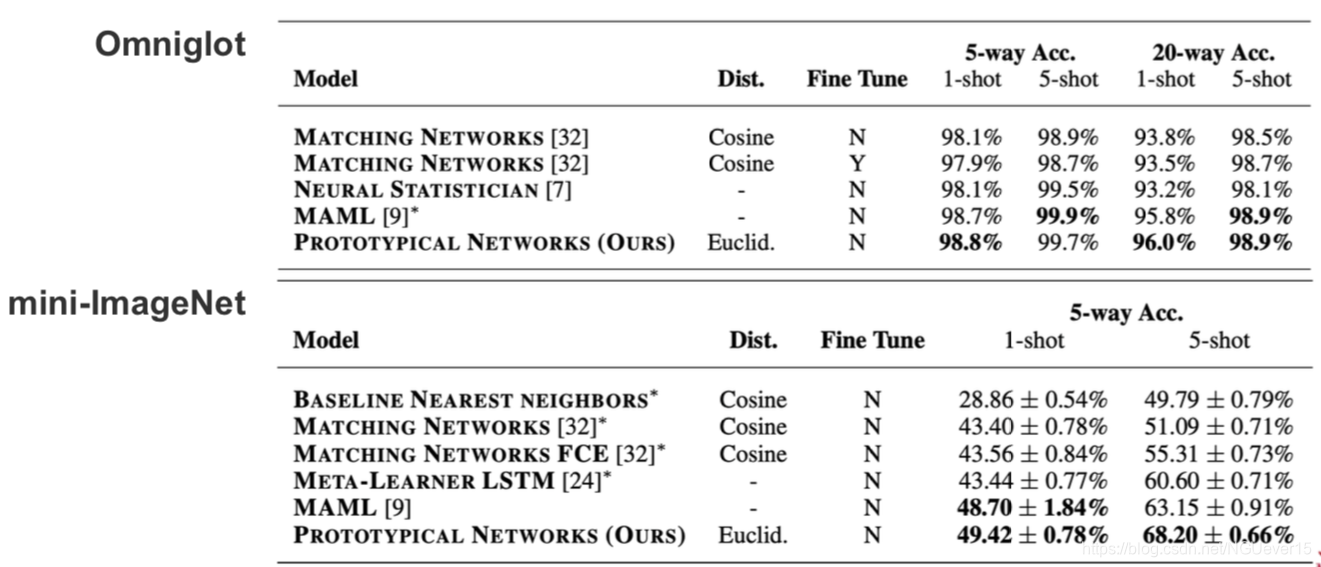
Rapid Learning or Feature Reuse 特征重用
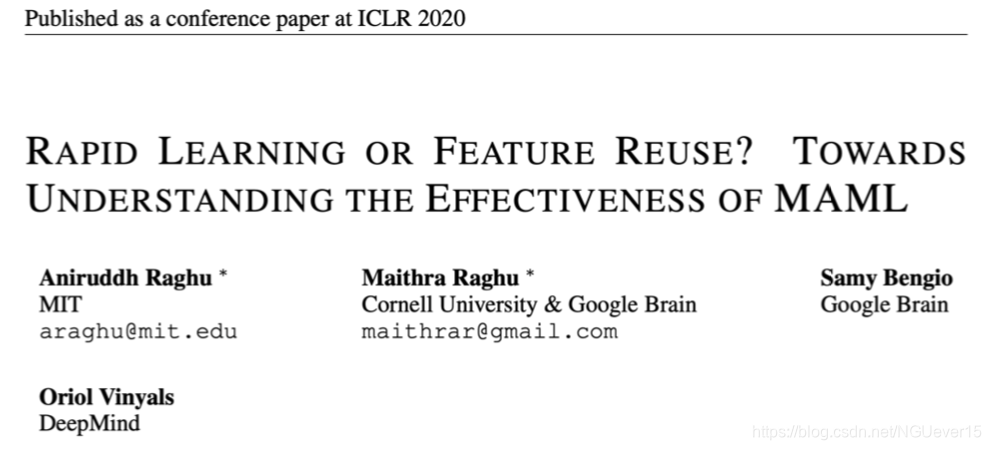
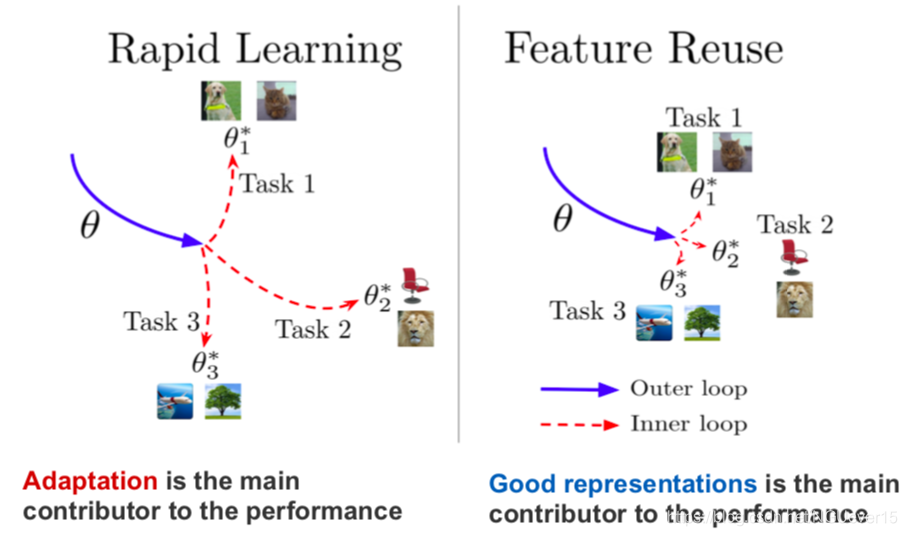
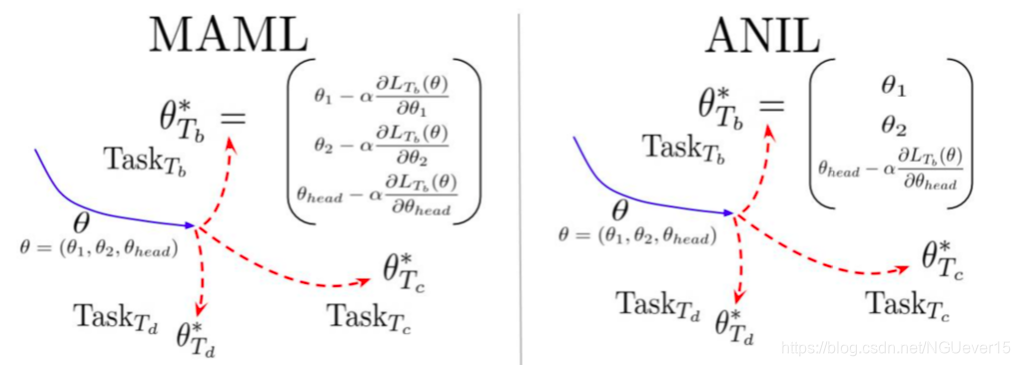
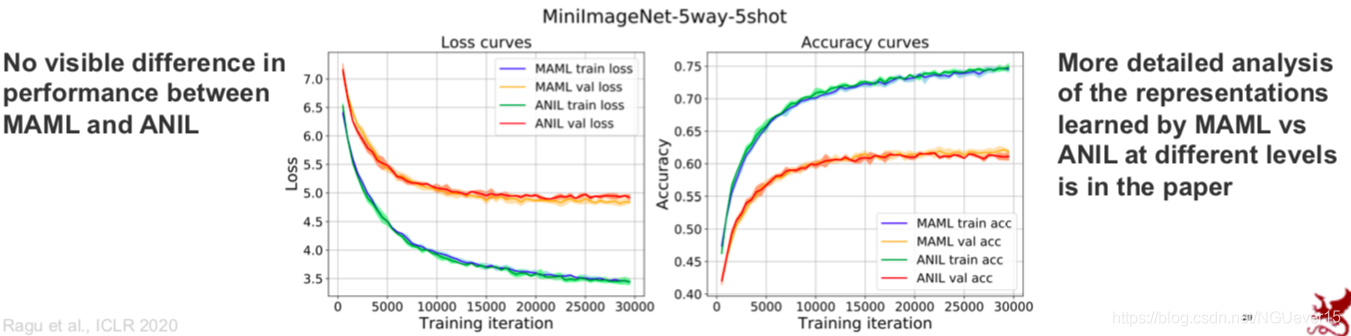
Drawing parallels between meta-learning and GPs
In few-shot learning:
- Learn to identify functions that generated the data from just a few examples.
- The function class and the adaptation rule encapsulate our prior knowledge.
Recall Gaussian Processes (GPs): 高斯过程
- Given a few (x, y) pairs, we can compute the predictive mean and variance.
- Our prior knowledge is encapsulated in the kernel function.
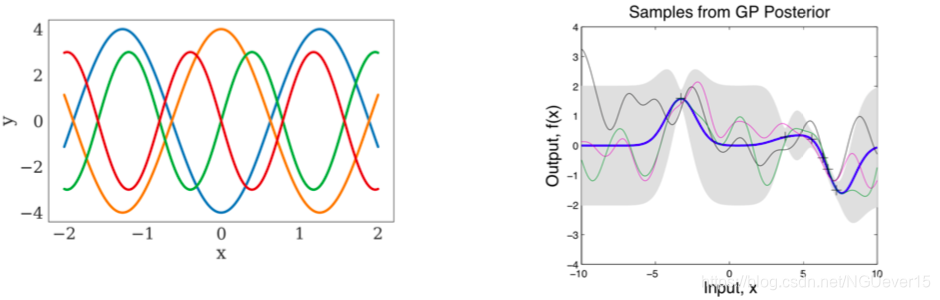
Conditional Neural Processes 条件神经过程
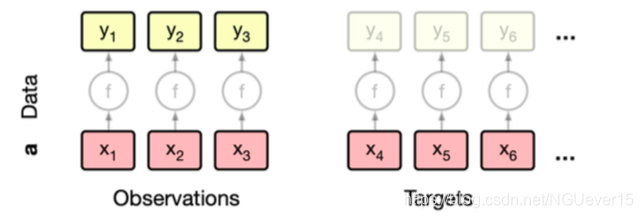
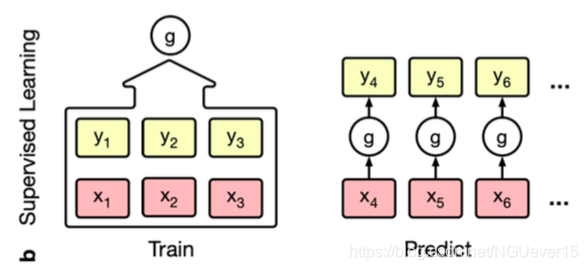
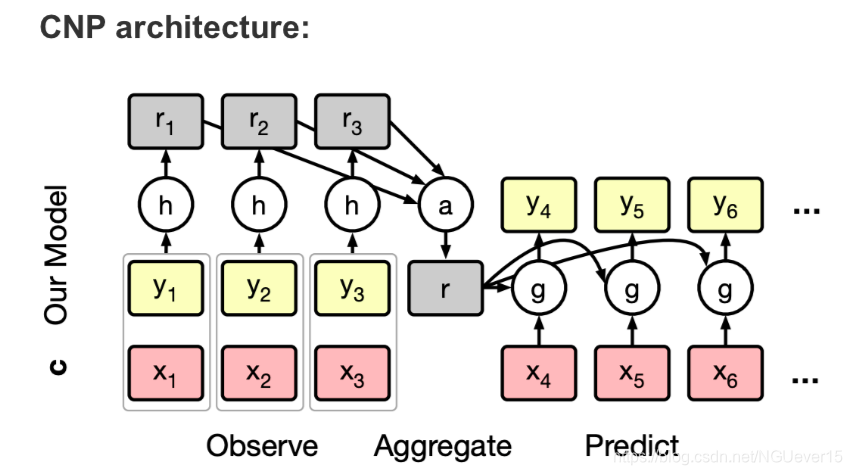
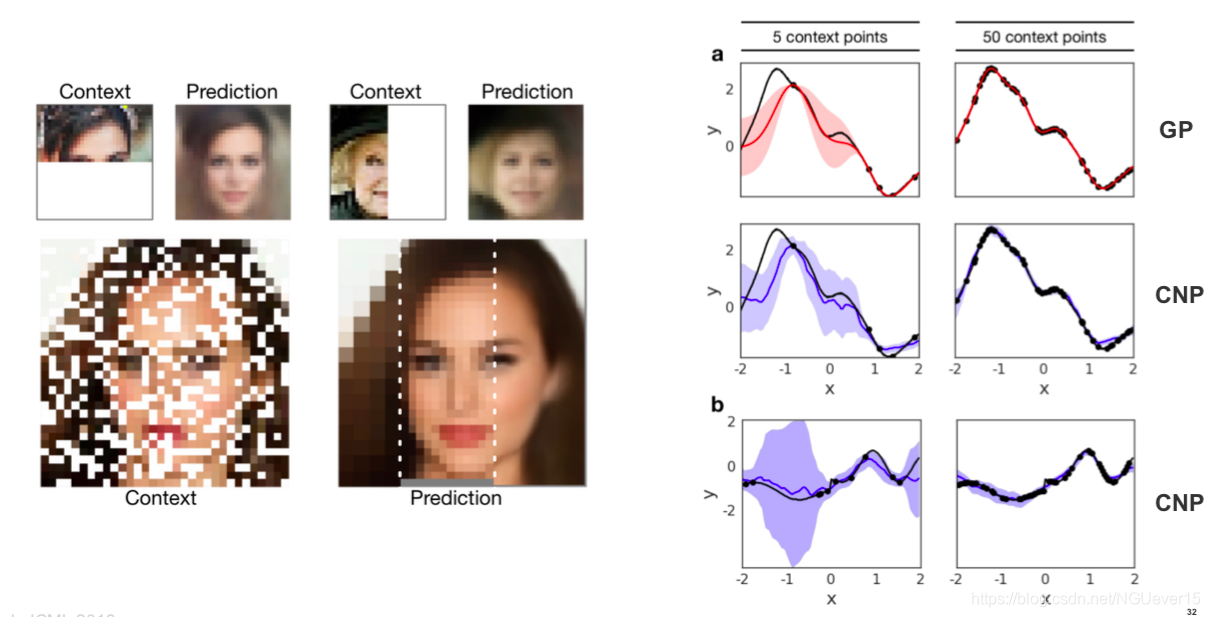
On software packages for meta-learning
A lot of research code releases (code is fragile and sometimes broken)
A few notable libraries that implement a few specific methods:
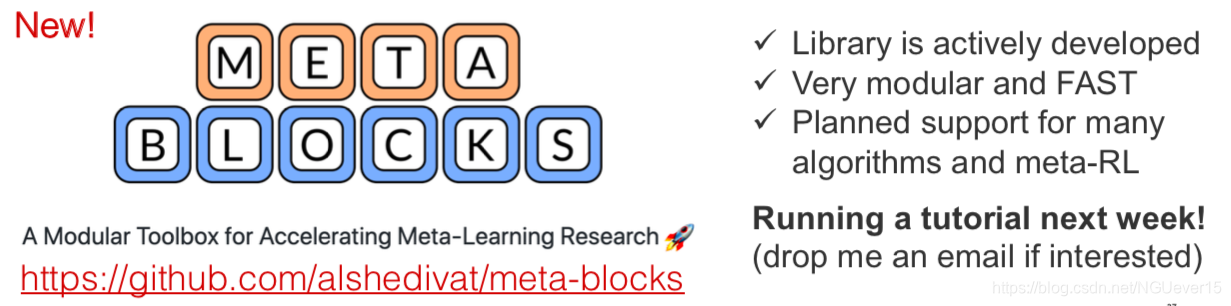
Takeaways
- Many real-world scenarios require building adaptive systems and cannot be solved using “learn-once” standard ML approach.
- Learning-to-learn (or meta-learning) attempts extend ML to rich multitask scenarios—instead of learning a function, learn a learning algorithm.
- Two families of widely popular methods:
- Gradient-based meta-learning (MAML and such)
- Prototype-based meta-learning (Protonets, Neural Processes, ...)
- Many hybrids, extensions, improvements (CAIVA, MetaSGD, ...)
- Is it about adaptation or learning good representations? Still unclear and depends on the task; having good representations might be enough.
- Meta-learning can be used as a mechanism for causal discovery.因果发现 (See Bengio et al., 2019.)
Recall the definition of learning-to-learn
Standard learning: Given a distribution over examples (single task), learn a function that minimizes the loss:

Learning-to-learn: Given a distribution over tasks, output an adaptation rule that can be used at test time to generalize from a task description

Meta reinforcement learning (RL): Given a distribution over environments, train a policy update rule that can solve new environments given only limited or no initial experience.
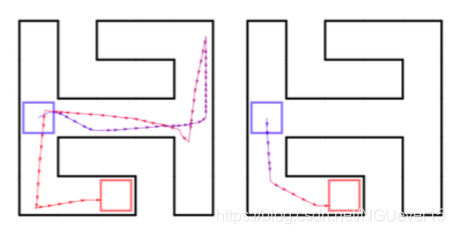
Meta-learning for RL
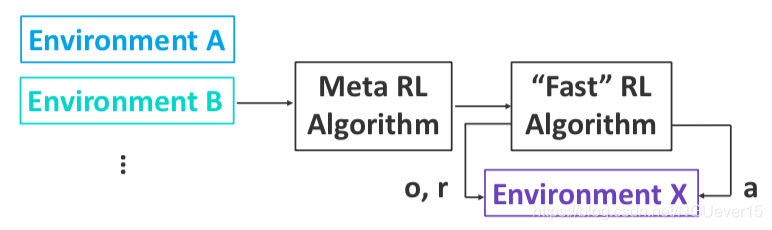
On-policy RL: Quick Recap 符合策略的RL:快速回顾
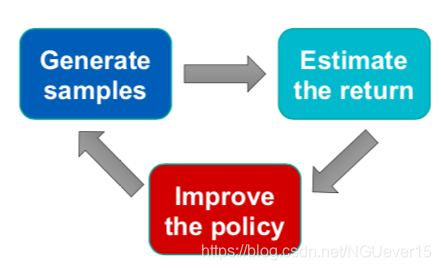
REINFORCE algorithm:
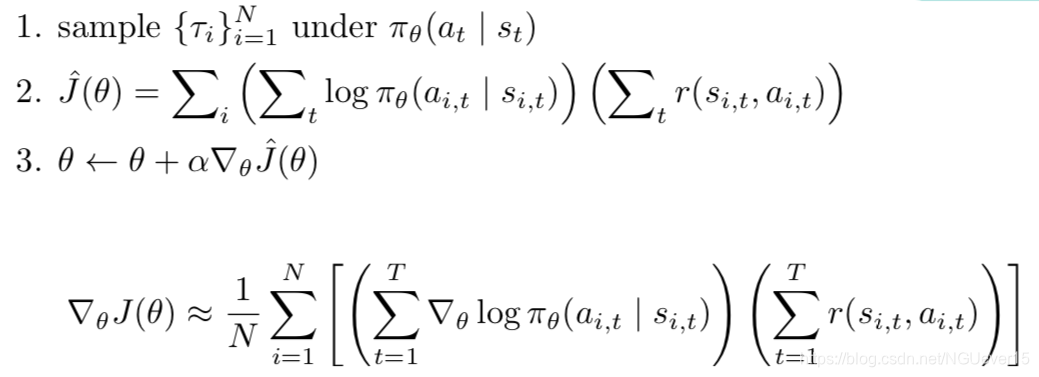
On-policy Meta-RL: MAML (again!)
- Start with a common policy initialization \(\theta\)
- Given a new task \(T_i\) , collect data using initial policy, then adapt using a gradient step:

- Meta-training is learning a shared initialization for all tasks:

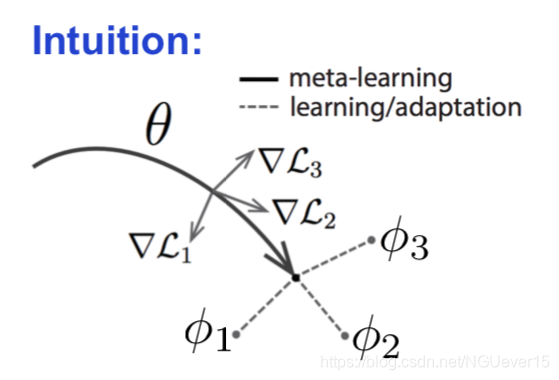
Adaptation as Inference 适应推理
Treat policy parameters, tasks, and all trajectories as random variables随机变量
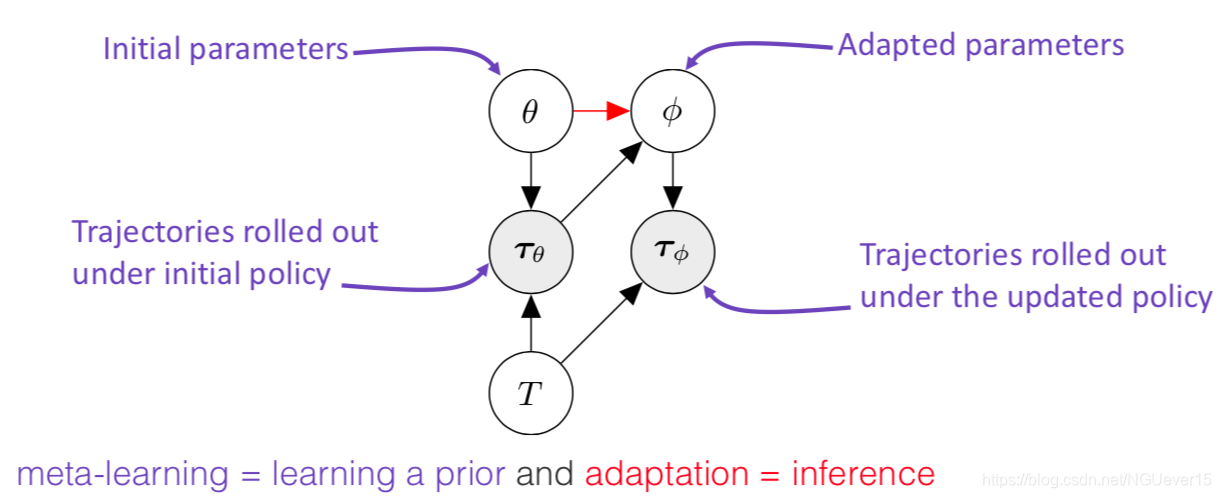
meta-learning = learning a prior and adaptation = inference
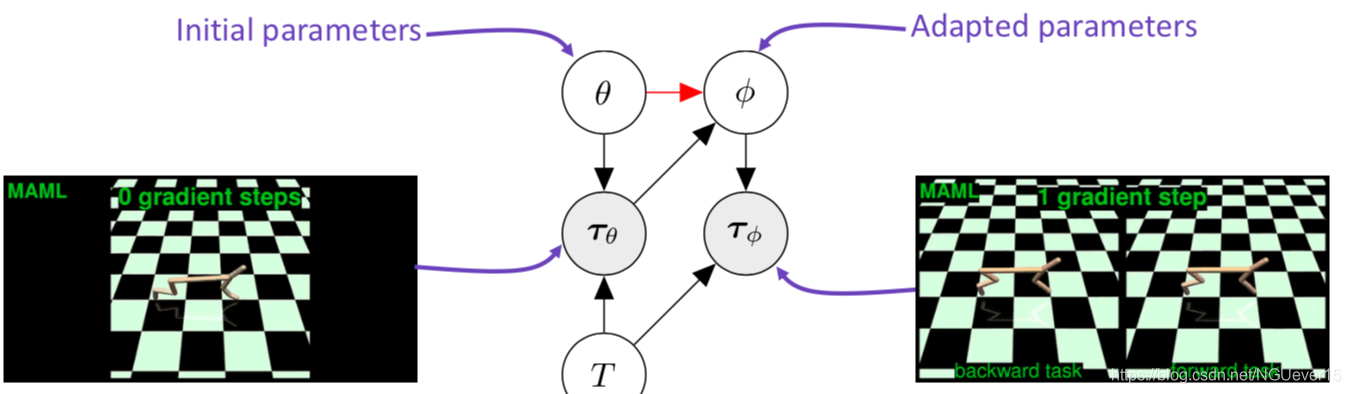
Off-policy meta-RL: PEARL
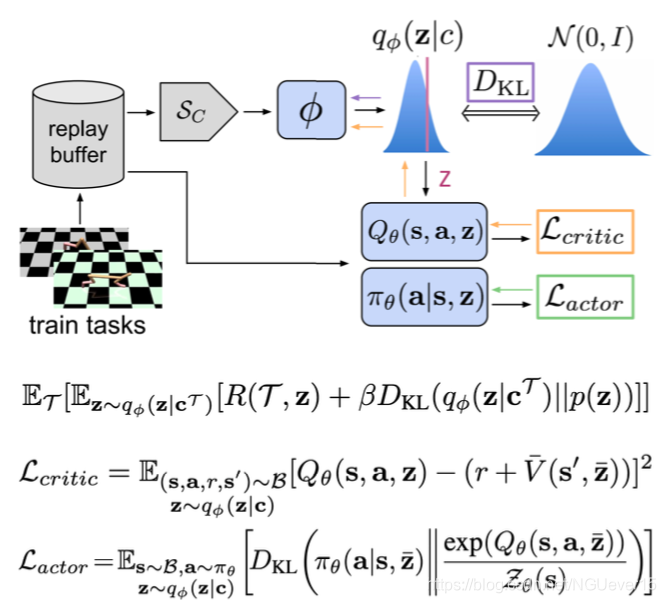
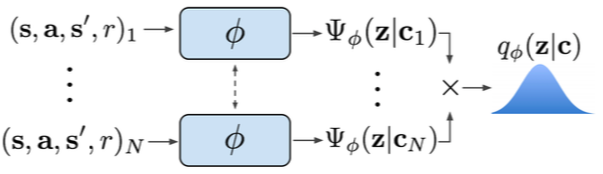
Key points:
- Infer latent representations z of each task from the trajectory data.
- The inference networkq is decoupled from the policy, which enables off-policy learning.
- All objectives involve the inference and policy networks.
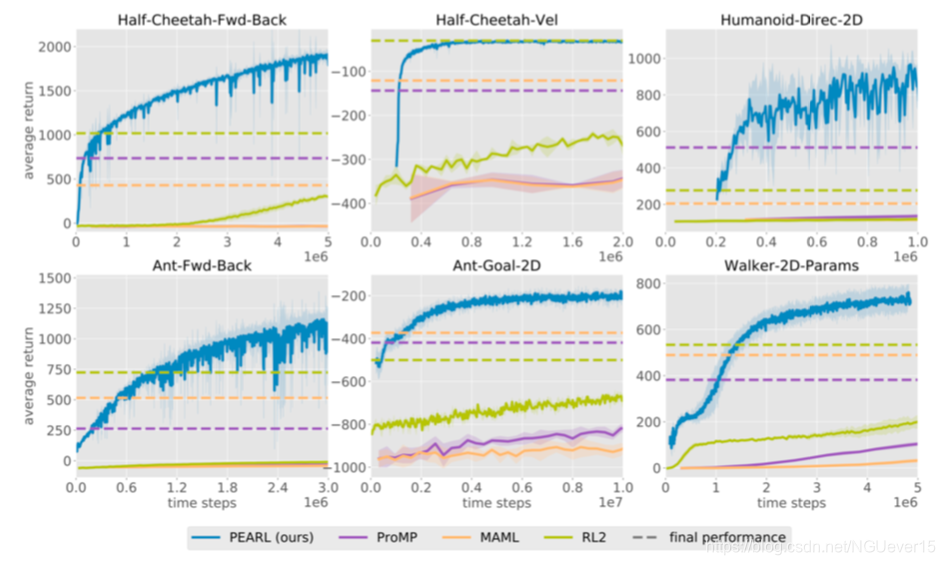
Adaptation in nonstationary environments 不稳定环境
Classical few-shot learning setup:
- The tasks are i.i.d. samples from some underlying distribution.
- Given a new task, we get to interact with it before adapting.
- What if we are in a nonstationary environment (i.e. changing over time)? Can we still use meta-learning?
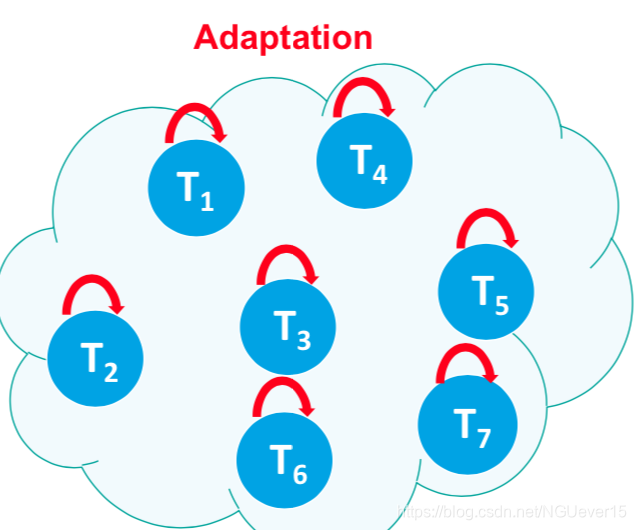
Example: adaptation to a learning opponent
Each new round is a new task. Nonstationary environment is a sequence of tasks.
Continuous adaptation setup:
- The tasks are sequentially dependent.
- meta-learn to exploit dependencies
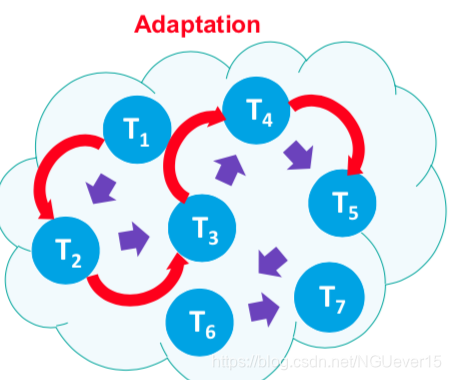
Continuous adaptation
Treat policy parameters, tasks, and all trajectories as random variables
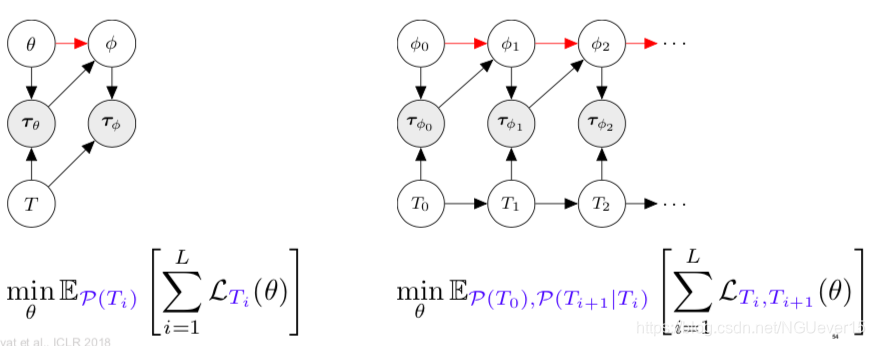
RoboSumo: a multiagent competitive env
an agent competes vs. an opponent, the opponent’s behavior changes over time
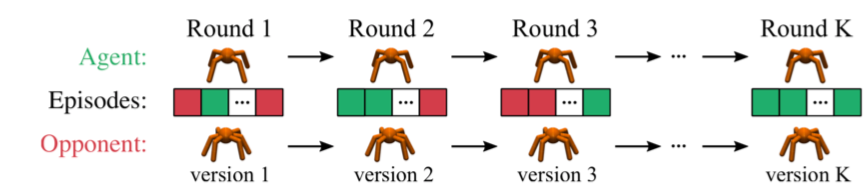
Takeaways
- Learning-to-learn (or meta-learning) setup is particularly suitable for multi-task reinforcement learning
- Both on-policy and off-policy RL can be “upgraded” to meta-RL:
- On-policy meta-RL is directly enabled by MAML
- Decoupling task inference and policy learning enables off-policy methods
- Is it about fast adaptation or learning good multitask representations? (See discussion in Meta-Q-Learning: https://arxiv.org/abs/1910.00125)
- Probabilistic view of meta-learning allows to use meta-learning ideas beyond distributions of i.i.d. tasks, e.g., continuous adaptation.
- Very active area of research.
卡耐基梅隆大学(CMU)元学习和元强化学习课程 | Elements of Meta-Learning
标签:can multitask change code chm nts -o init sdn
原文地址:https://www.cnblogs.com/joselynzhao/p/12892696.html