标签:
1:
神经网络中,我们通过最小化神经网络来训练网络,所以在训练时最后一层是损失函数层(LOSS),
在测试时我们通过准确率来评价该网络的优劣,因此最后一层是准确率层(ACCURACY)。
但是当我们真正要使用训练好的数据时,我们需要的是网络给我们输入结果,对于分类问题,我们需要获得分类结果,如下右图最后一层我们得到
的是概率,我们不需要训练及测试阶段的LOSS,ACCURACY层了。
下图是能过$CAFFE_ROOT/python/draw_net.py绘制$CAFFE_ROOT/models/caffe_reference_caffnet/train_val.prototxt , $CAFFE_ROOT/models/caffe_reference_caffnet/deploy.prototxt,分别代表训练时与最后使用时的网络结构。
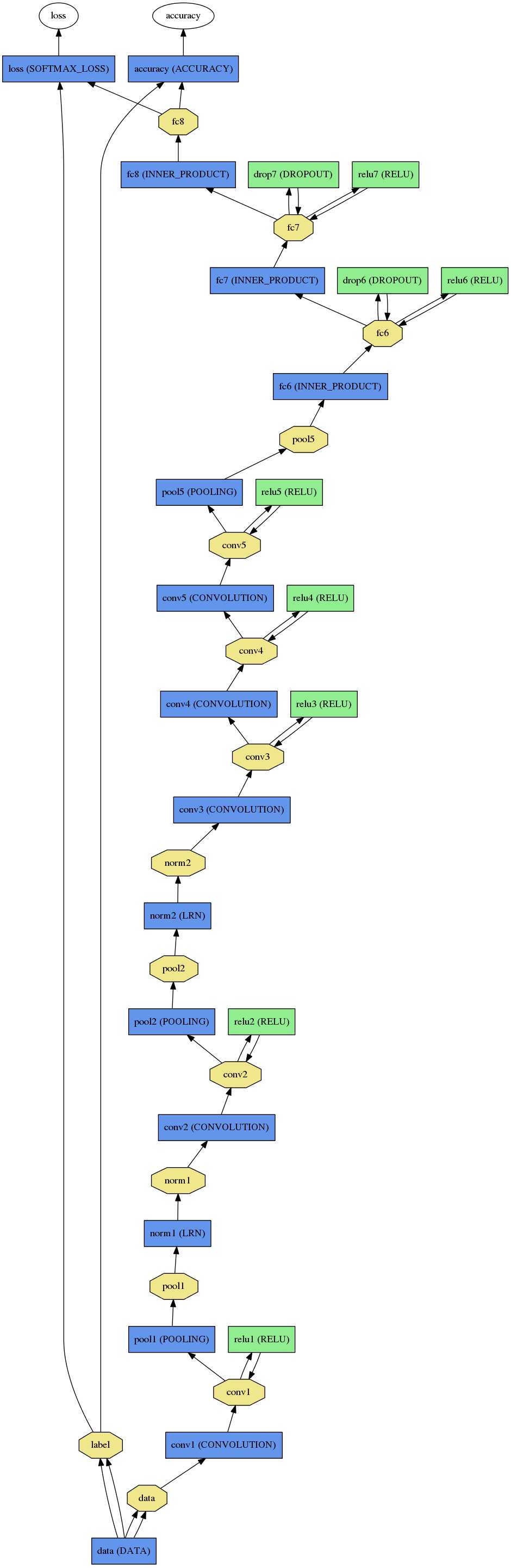
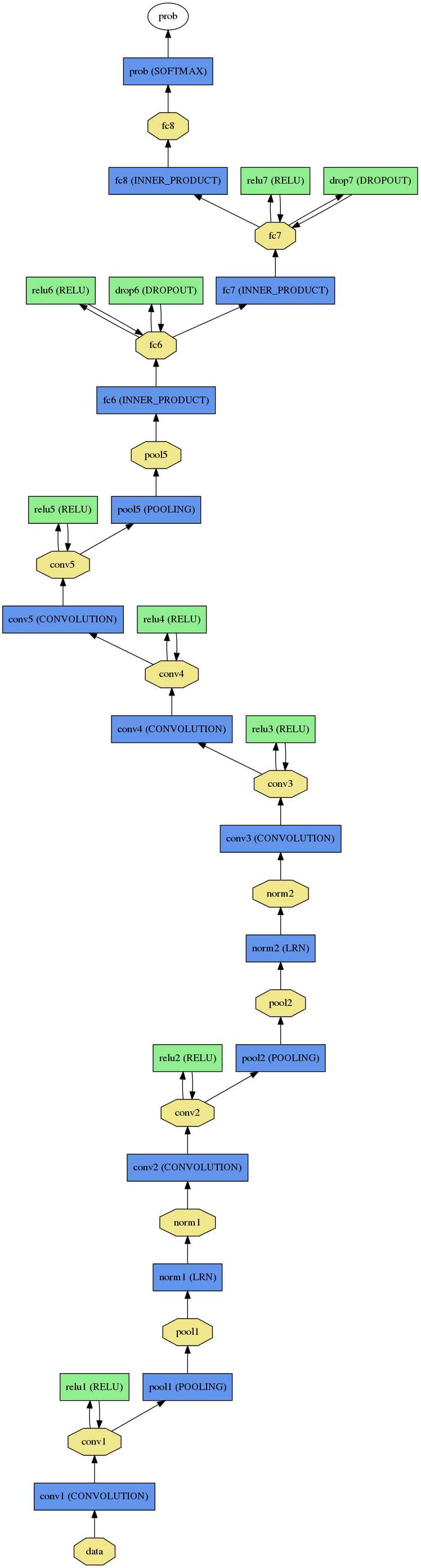
我们一般将train与test放在同一个.prototxt中,需要在data层输入数据的source,
而在使用时.prototxt只需要定义输入图片的大小通道数据参数即可,如下图所示,分别是
$CAFFE_ROOT/models/caffe_reference_caffnet/train_val.prototxt , $CAFFE_ROOT/models/caffe_reference_caffnet/deploy.prototxt的data层
训练时, solver.prototxt中使用的是rain_val.prototxt
./build/tools/caffe/train -solver ./models/bvlc_reference_caffenet/solver.prototxt
使用上面训练的网络提取特征,使用的网络模型是deploy.prototxt
./build/tools/extract_features.bin models/bvlc_refrence_caffenet.caffemodel models/bvlc_refrence_caffenet/deploy.prototxt
。。
2:
(1)介绍 *_train_test.prototxt文件与 *_deploy.prototxt文件的不http://blog.csdn.net/sunshine_in_moon/article/details/49472901
(2)生成deploy文件的Python代码:http://www.cnblogs.com/denny402/p/5685818.html
*_train_test.prototxt文件:这是训练与测试网络配置文件
*_deploy.prototxt文件:这是模型构造文件
在博文http://www.cnblogs.com/denny402/p/5685818.html 中给出了生成 deploy.prototxt文件的Python源代码,但是每个网络不同,修改起来比较麻烦,下面给出该博文中以mnist为例生成deploy文件的源代码,可根据自己网络的设置做出相应修改:(下方代码未测试)
# -*- coding: utf-8 -*-
from caffe import layers as L,params as P,to_proto
root=‘/home/xxx/‘
deploy=root+‘mnist/deploy.prototxt‘ #文件保存路径
def create_deploy():
#少了第一层,data层
conv1=L.Convolution(bottom=‘data‘, kernel_size=5, stride=1,num_output=20, pad=0,weight_filler=dict(type=‘xavier‘))
pool1=L.Pooling(conv1, pool=P.Pooling.MAX, kernel_size=2, stride=2)
conv2=L.Convolution(pool1, kernel_size=5, stride=1,num_output=50, pad=0,weight_filler=dict(type=‘xavier‘))
pool2=L.Pooling(conv2, pool=P.Pooling.MAX, kernel_size=2, stride=2)
fc3=L.InnerProduct(pool2, num_output=500,weight_filler=dict(type=‘xavier‘))
relu3=L.ReLU(fc3, in_place=True)
fc4 = L.InnerProduct(relu3, num_output=10,weight_filler=dict(type=‘xavier‘))
#最后没有accuracy层,但有一个Softmax层
prob=L.Softmax(fc4)
return to_proto(prob)
def write_deploy():
with open(deploy, ‘w‘) as f:
f.write(‘name:"Lenet"\n‘)
f.write(‘input:"data"\n‘)
f.write(‘input_dim:1\n‘)
f.write(‘input_dim:3\n‘)
f.write(‘input_dim:28\n‘)
f.write(‘input_dim:28\n‘)
f.write(str(create_deploy()))
if __name__ == ‘__main__‘:
write_deploy()
用代码生成deploy文件还是比较麻烦。我们在构建深度学习网络时,肯定会先定义好训练与测试网络的配置文件——*_train_test.prototxt文件,我们可以通过修改*_train_test.prototxt文件 来生成 deploy 文件。以cifar10为例先简单介绍一下两者的区别。
(1)deploy 文件中的数据层更为简单,即将*_train_test.prototxt文件中的输入训练数据lmdb与输入测试数据lmdb这两层删除,取而代之的是,
- layer {
- name: "data"
- type: "Input"
- top: "data"
- input_param { shape: { dim: 1 dim: 3 dim: 32 dim: 32 } }
- }
注:shape: { dim: 1 dim: 3 dim: 32 dim: 32 }代表含义:
shape {
dim: 1 #num,可自行定义
dim: 3 #通道数,表示RGB三个通道
dim: 32 #图像的长和宽,通过 *_train_test.prototxt文件中数据输入层的crop_size获取
dim: 32
(2)卷积层和全连接层中weight_filler{}与bias_filler{}两个参数不用再填写,因为这两个参数的值,由已经训练好的模型*.caffemodel文件提供。如下所示代码,将*_train_test.prototxt文件中的weight_filler、bias_filler全部删除。
layer { # weight_filler、bias_filler删除
name: "ip2"
type: "InnerProduct"
bottom: "ip1" top: "ip2"
param {
lr_mult: 1 #权重w的学习率倍数
}
param { lr_mult: 2 #偏置b的学习率倍数
}
inner_product_param { num_output: 10
weight_filler { type: "gaussian" std: 0.1 }
bias_filler { type: "constant" }
}
}
删除后变为
- layer {
- name: "ip2"
- type: "InnerProduct"
- bottom: "ip1"
- top: "ip2"
- param {
- lr_mult: 1
- }
- param {
- lr_mult: 2
- }
- inner_product_param {
- num_output: 10
- }
- }
(3)输出层的变化
1)没有了test模块测试精度 ,将该层删除
2)输出层
1)*_deploy.prototxt文件的构造和*_train_test.prototxt文件的构造最为明显的不同点是,deploy文件没有test网络中的test模块,只有训练模块,即将*_train_test.prototxt中最后部分的test模块测试精度删除,即将如下代码删除。
- layer {
- name: "accuracy"
- type: "Accuracy"
- bottom: "ip2"
- bottom: "label"
- top: "accuracy"
- include {
- phase: TEST
- }
- }
2) 输出层
*_train_test.prototxt文件
- layer{
- name: "loss"
- type: "SoftmaxWithLoss"
- bottom: "ip2"
- bottom: "label"
- top: "loss"
- }
*_deploy.prototxt文件
[python]
- layer {
- name: "prob"
- type: "Softmax"
- bottom: "ip2"
- top: "prob"
- }
注意在两个文件中输出层的类型都发生了变化一个是SoftmaxWithLoss,另一个是Softmax。另外为了方便区分训练与应用输出,训练是输出时是loss,应用时是prob。
下面给出CIFAR10中的配置文件cifar10_quick_train_test.prototxt与其模型构造文件 cifar10_quick.prototxt 直观展示两者的区别。
cifar10_quick_train_test.prototxt文件代码
cifar10_quick_train_test.prototxt文件代码
name: "CIFAR10_quick"
layer { #该层去掉
name: "cifar"
type: "Data"
top: "data"
top: "label"
include {
phase: TRAIN
}
transform_param {
mean_file: "examples/cifar10/mean.binaryproto"
}
data_param {
source: "examples/cifar10/cifar10_train_lmdb"
batch_size: 100
backend: LMDB
}
}
layer { #该层去掉
name: "cifar"
type: "Data"
top: "data"
top: "label"
include {
phase: TEST
}
transform_param {
mean_file: "examples/cifar10/mean.binaryproto"
}
data_param {
source: "examples/cifar10/cifar10_test_lmdb"
batch_size: 100
backend: LMDB
}
}
layer { #将下方的weight_filler、bias_filler全部删除
name: "conv1"
type: "Convolution"
bottom: "data"
top: "conv1"
param {
lr_mult: 1
}
param {
lr_mult: 2
}
convolution_param {
num_output: 32
pad: 2
kernel_size: 5
stride: 1
weight_filler {
type: "gaussian"
std: 0.0001
}
bias_filler {
type: "constant"
}
}
}
layer {
name: "pool1"
type: "Pooling"
bottom: "conv1"
top: "pool1"
pooling_param {
pool: MAX
kernel_size: 3
stride: 2
}
}
layer {
name: "relu1"
type: "ReLU"
bottom: "pool1"
top: "pool1"
}
layer { #weight_filler、bias_filler删除
name: "conv2"
type: "Convolution"
bottom: "pool1"
top: "conv2"
param {
lr_mult: 1
}
param {
lr_mult: 2
}
convolution_param {
num_output: 32
pad: 2
kernel_size: 5
stride: 1
weight_filler {
type: "gaussian"
std: 0.01
}
bias_filler {
type: "constant"
}
}
}
layer {
name: "relu2"
type: "ReLU"
bottom: "conv2"
top: "conv2"
}
layer {
name: "pool2"
type: "Pooling"
bottom: "conv2"
top: "pool2"
pooling_param {
pool: AVE
kernel_size: 3
stride: 2
}
}
layer { #weight_filler、bias_filler删除
name: "conv3"
type: "Convolution"
bottom: "pool2"
top: "conv3"
param {
lr_mult: 1
}
param {
lr_mult: 2
}
convolution_param {
num_output: 64
pad: 2
kernel_size: 5
stride: 1
weight_filler {
type: "gaussian"
std: 0.01
}
bias_filler {
type: "constant"
}
}
}
layer {
name: "relu3"
type: "ReLU"
bottom: "conv3"
top: "conv3"
}
layer {
name: "pool3"
type: "Pooling"
bottom: "conv3"
top: "pool3"
pooling_param {
pool: AVE
kernel_size: 3
stride: 2
}
}
layer { #weight_filler、bias_filler删除
name: "ip1"
type: "InnerProduct"
bottom: "pool3"
top: "ip1"
param {
lr_mult: 1
}
param {
lr_mult: 2
}
inner_product_param {
num_output: 64
weight_filler {
type: "gaussian"
std: 0.1
}
bias_filler {
type: "constant"
}
}
}
layer { # weight_filler、bias_filler删除
name: "ip2"
type: "InnerProduct"
bottom: "ip1"
top: "ip2"
param {
lr_mult: 1
}
param {
lr_mult: 2
}
inner_product_param {
num_output: 10
weight_filler {
type: "gaussian"
std: 0.1
}
bias_filler {
type: "constant"
}
}
}
layer { #将该层删除
name: "accuracy"
type: "Accuracy"
bottom: "ip2"
bottom: "label"
top: "accuracy"
include {
phase: TEST
}
}
layer { #修改
name: "loss" #---loss 修改为 prob
type: "SoftmaxWithLoss" # SoftmaxWithLoss 修改为 softmax
bottom: "ip2"
bottom: "label" #去掉
top: "loss"
}
以下为cifar10_quick.prototxt
layer { #将两个输入层修改为该层
name: "data"
type: "Input"
top: "data"
input_param { shape: { dim: 1 dim: 3 dim: 32 dim: 32 } } #注意shape中变量值的修改,CIFAR10中的 *_train_test.protxt文件中没有 crop_size
}
layer {
name: "conv1"
type: "Convolution"
bottom: "data"
top: "conv1"
param {
lr_mult: 1 #权重W的学习率倍数
}
param {
lr_mult: 2 #偏置b的学习率倍数
}
convolution_param {
num_output: 32
pad: 2 #加边为2
kernel_size: 5
stride: 1
}
}
layer {
name: "pool1"
type: "Pooling"
bottom: "conv1"
top: "pool1"
pooling_param {
pool: MAX #Max Pooling
kernel_size: 3
stride: 2
}
}
layer {
name: "relu1"
type: "ReLU"
bottom: "pool1"
top: "pool1"
}
layer {
name: "conv2"
type: "Convolution"
bottom: "pool1"
top: "conv2"
param {
lr_mult: 1
}
param {
lr_mult: 2
}
convolution_param {
num_output: 32
pad: 2
kernel_size: 5
stride: 1
}
}
layer {
name: "relu2"
type: "ReLU"
bottom: "conv2"
top: "conv2"
}
layer {
name: "pool2"
type: "Pooling"
bottom: "conv2"
top: "pool2"
pooling_param {
pool: AVE #均值池化
kernel_size: 3
stride: 2
}
}
layer {
name: "conv3"
type: "Convolution"
bottom: "pool2"
top: "conv3"
param {
lr_mult: 1
}
param {
lr_mult: 2
}
convolution_param {
num_output: 64
pad: 2
kernel_size: 5
stride: 1
}
}
layer {
name: "relu3"
type: "ReLU" #使用ReLU激励函数,这里需要注意的是,本层的bottom和top都是conv3>
bottom: "conv3"
top: "conv3"
}
layer {
name: "pool3"
type: "Pooling"
bottom: "conv3"
top: "pool3"
pooling_param {
pool: AVE
kernel_size: 3
stride: 2
}
}
layer {
name: "ip1"
type: "InnerProduct"
bottom: "pool3"
top: "ip1"
param {
lr_mult: 1
}
param {
lr_mult: 2
}
inner_product_param {
num_output: 64
}
}
layer {
name: "ip2"
type: "InnerProduct"
bottom: "ip1"
top: "ip2"
param {
lr_mult: 1
}
param {
lr_mult: 2
}
inner_product_param {
num_output: 10
}
}
layer {
name: "prob"
type: "Softmax"
bottom: "ip2"
top: "prob"
}
3:
将train_val.prototxt 转换成deploy.prototxt
1.删除输入数据(如:type:data...inckude{phase: TRAIN}),然后添加一个数据维度描述。
- input: "data"
- input_dim: 1
- input_dim: 3
- input_dim: 224
- input_dim: 224
- force_backward: true
2.移除最后的“loss” 和“accuracy” 层,加入“prob”层。
[plain]
- layers {
- name: "prob"
- type: SOFTMAX
- bottom: "fc8"
- top: "prob"
- }
如果train_val文件中还有其他的预处理层,就稍微复杂点。如下,在‘data‘层,在‘data’层和‘conv1’层(with bottom:”data” / top:”conv1″). 插入一个层来计算输入数据的均值。
- layer {
- name: “mean”
- type: “Convolution”
- <strong>bottom: “data”
- top: “data”</strong>
- param {
- lr_mult: 0
- decay_mult: 0
- }
-
- …}
在deploy.prototxt文件中,“mean” 层必须保留,只是容器改变,相应的‘conv1’也要改变 ( bottom:”mean”/ top:”conv1″ )。
[plain]
- layer {
- name: “mean”
- type: “Convolution”
- <strong>bottom: “data”
- top: “mean“</strong>
- param {
- lr_mult: 0
- decay_mult: 0
- }
-
- …}
4:
生成deploy文件
如果要把训练好的模型拿来测试新的图片,那必须得要一个deploy.prototxt文件,这个文件实际上和test.prototxt文件差不多,只是头尾不相同而也。deploy文件没有第一层数据输入层,也没有最后的Accuracy层,但最后多了一个Softmax概率层。
这里我们采用代码的方式来自动生成该文件,以mnist为例。
deploy.py
# -*- coding: utf-8 -*-
from caffe import layers as L,params as P,to_proto
root=‘/home/xxx/‘
deploy=root+‘mnist/deploy.prototxt‘ #文件保存路径
def create_deploy():
#少了第一层,data层
conv1=L.Convolution(bottom=‘data‘, kernel_size=5, stride=1,num_output=20, pad=0,weight_filler=dict(type=‘xavier‘))
pool1=L.Pooling(conv1, pool=P.Pooling.MAX, kernel_size=2, stride=2)
conv2=L.Convolution(pool1, kernel_size=5, stride=1,num_output=50, pad=0,weight_filler=dict(type=‘xavier‘))
pool2=L.Pooling(conv2, pool=P.Pooling.MAX, kernel_size=2, stride=2)
fc3=L.InnerProduct(pool2, num_output=500,weight_filler=dict(type=‘xavier‘))
relu3=L.ReLU(fc3, in_place=True)
fc4 = L.InnerProduct(relu3, num_output=10,weight_filler=dict(type=‘xavier‘))
#最后没有accuracy层,但有一个Softmax层
prob=L.Softmax(fc4)
return to_proto(prob)
def write_deploy():
with open(deploy, ‘w‘) as f:
f.write(‘name:"Lenet"\n‘)
f.write(‘input:"data"\n‘)
f.write(‘input_dim:1\n‘)
f.write(‘input_dim:3\n‘)
f.write(‘input_dim:28\n‘)
f.write(‘input_dim:28\n‘)
f.write(str(create_deploy()))
if __name__ == ‘__main__‘:
write_deploy()
运行该文件后,会在mnist目录下,生成一个deploy.prototxt文件。
这个文件不推荐用代码来生成,反而麻烦。大家熟悉以后可以将test.prototxt复制一份,修改相应的地方就可以了,更加方便。
5:
Convert train_val.prototxt to deploy.prototxt
- Remove input datalayer and insert a description of input data dimension
- Remove “loss” and “accuracy” layer and insert “prob” layer at the end
Here is a google groups link.
If you have preprocessing layers, things get a bit more tricky.
For example, in train_val.prototxt, which includes the “data” layer, I insert a layer to calculate the mean over the channels of input data,
layer { name: “mean” type: “Convolution” bottom: “data” top: “data” param { lr_mult: 0 decay_mult: 0 }
…}
between “data” layer and “conv1” layer (with bottom:”data” / top:”conv1″).
In deploy.prototxt, the “mean” layer has to be retained, yet its container needs to be changed! i.e.
layer { name: “mean” type: “Convolution” bottom: “data” top: “mean“ param { lr_mult: 0 decay_mult: 0 }
…}
and the “conv1” layer needs to be changed accordingly, ( bottom:”mean”/ top:”conv1″ ).
It’s fine to use train_val.prototxt with “mean” layer using “data” container in the training phase, and use deploy.prototxt with “mean” layer using “mean” container in the testing phase in python. The learned caffemodel can be loaded correctly.
4'.deploy.prototxt
标签:
原文地址:http://www.cnblogs.com/carle-09/p/5779555.html